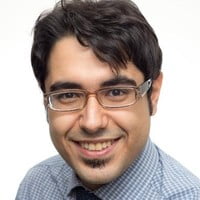
Foad Sohrabi
Artificial intelligence (AI) and machine learning (ML) methods have recently shown great potential in dealing with complex and high-dimensional optimization problems in various wireless communications settings. By leveraging the concept of big data, AI/ML approaches can provide robust performance with reasonable computational complexity for designing large-scale systems. Accordingly, AI/ML approaches have advantages from both performance and complexity perspectives over conventional optimization-based methods, especially for scenarios in which channel models are not accurate, the problem formulation is complicated, or there is a non-linearity in the system. Motivated by such nice properties of AI/ML methods, the 3rd generation partnership project (3GPP) has just started investigating the application of AI/ML for NR air interface in its latest release (Rel. 18). As initial use cases, 3GPP has identified the following three applications: (i) channel state information (CSI) feedback enhancement, (ii) beam management, and (iii) positioning accuracy enhancement. This post briefly introduces each of these three applications.
CSI feedback enhancement: Massive multiple-input multiple-output (MIMO) technology in which the base station (BS) is equipped with a massive number of antennas is one of the key enablers for 5G and beyond. To exploit the advantages of massive MIMO systems, an accurate downlink channel state information (CSI) should be acquired at the BS to optimally design the multiuser beamformers and hence to fully utilize the spatial diversity and multiplexing gains provided by massive MIMO. In the FDD operation mode in which channel reciprocity assumption does not hold, the CSI is first obtained at the user equipment (UE), then it is fed back to the BS. Since in the massive MIMO regime, channels are high dimensional, the overhead of CSI feedback can be significant. Accordingly, the UE must compress the downlink CSI locally and then to feed a compressed version of the CSI back to the BS in massive MIMO systems. Such a CSI acquisition procedure can be perfectly modeled by an auto-encoder consisting of an encoder at the UE and a decoder at the BS. In particular, the estimated channel matrix at the UE is fed into a deep neural network (DNN) encoder to be mapped to a low-dimensional quantized signal. The compressed signal is then sent back to the BS via the uplink feedback channel and the BS finally reconstructs the CSI by employing a DNN decoder. In such an auto-encoder, the goal of the DNNs is to capture existing spatial, frequency-domain, and time-domain correlations in the channel matrix. Accordingly, the neural network architectures that have been widely used in machine learning literature for capturing spatial correlations, such as convolutional neural networks (CNNs), or for capturing temporal correlations, such as recurrent neural networks (RNNs), are great candidates to be employed in the described CSI feedback autoencoder.
Beam management: The idea of mmWave massive MIMO communication is typically implemented through analog or hybrid beamforming architectures to reduce the number of radio-frequency (RF) chains, thus lowering power consumption and hardware cost. In such an RF chain limited scenario, instead of estimating high-dimensional channels for beamforming design purposes, a beam management procedure is performed to find the best transmitter-receiver beam pair. The conventional beam management approach is based on exhaustive beam sweeping. Although exhaustive beam sweeping can achieve excellent performance, it leads to significant time delay and power consumption. To alleviate such drawbacks of exhaustive beam sweeping, sparse beam sweeping has been introduced in which a beam pair is selected by adopting iterative beam search strategies. However, the existing algorithms developed for sparse beam sweeping are far from optimal, especially in higher frequency bands (FR2) and with high-speed UEs. Now, thanks to the advent of data-driven AI/ML methods, we can exploit the historical information in the training data set to construct a mapping function from sparse beam sweeping measurements to the best beam pair.
Positioning accuracy enhancement: Accurate positioning is a crucial component in several 5G industrial internet of things (IoT) use cases and verticals such as smart factories. The conventional model-based positioning approaches are based on explicit mapping functions from timing or angle measurements to the user’s location. Such mapping functions are mainly developed for scenarios with multiple line-of-sight (LoS) paths between the target and multiple (transmission-reception points) TRPs. However, in most practical industrial settings, the radio signals experience non-line-of-sight (NLoS) conditions due to the existence of metallic objects with large and irregular sizes. So, it is very important to develop new positioning algorithms for scenarios with an extremely high probability of being NLoS. AI/ML-based methods are promising solutions for such a challenging localization task since AI/ML methods can simply learn an acceptable mapping function from the measurements to the UE positions by exploiting the labeled data in the training phase.
In summary, the integration of AI technologies in future wireless networks will empower them to tackle challenging physical-layer problems that conventional model-based approaches fail to solve efficiently. However, to employ AI/ML frameworks in practical wireless communication systems, we need to comprehensively study the involved practical challenges including robustness, generalizability, interpretability, and training complexity. At Ofinno, the 5G research team has been conducting research on these practical aspects of using AI/ML for NR air interface and investigating the potential specification impact of adopting such data-driven methods.
Looking to supercharge your R&D and bolster your IP? Click here.